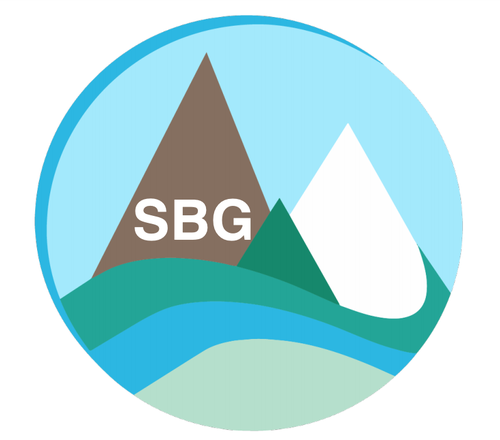
NASA is planning a hyperspectral space mission, Surface Biology and Geology (SBG) which is founded on findings of the National Academies of Sciences, Engineering and Medicine (NASEM) 2017 Decadal Survey titled Thriving on Our Changing Planet: A Decadal Strategy for Earth Observations from Space. http://sites.nationalacademies.org/DEPS/ESAS2017/index.htm
The decadal survey identified several ‘Very Important’ priorities, which stand to enhance the observational efficacy of Australian federal and state environmental agencies valuable ecological, hydrological and geological work. The most important priorities, and their relevance are highlighted below, and I have commented below each parallels with New South Wales Department of Climate Change, Energy, the Environment and Water (DCCEEW) Biodiversity program and other Commonwealth initiatives:
Ecosystems
E1a: Quantify the distribution of the functional traits, functional types, and composition of vegetation and marine biomass, spatially and over time.
- Plant traits are used to assess threatened species adaptive capacity.
- Plant Community Types (PCT) are key to the NSW Biodiversity Offsets Scheme and represent ‘composition of vegetation’. Thus, function and composition are key defining elements of PCT’s.
- Marine biomass, algae and cyanobacteria are ecological threats to our estuaries and waterways.
E1c: Quantify the physiological dynamics of terrestrial and aquatic primary producers.
- The health of our rivers, their aquatic ecology, baseline monitoring programs like UNE’s EcoHealth (https://ecohealth.une.edu.au) is an exemplar that focus on catchment scale assessment of aquatic ecosystems will be enhance by SBG’s data streams.
- Australia’s own CSIRO AquaWatch Australia program will provide a ‘Weather service for water quality’ that further enhances this very important priority for our water ways.
- International collaboration between Australia’s own HyVista Corporation (https://hyvista.com/ ) and the NASA AVIRIS team (https://aviris.jpl.nasa.gov/ ) on cal/val stands to further enhance high resolution hyperspectral (sub SBG pixel) data collection.
E2a: Quantify the fluxes of CO2 and CH4 globally at spatial scales of 100 to 500 km and monthly temporal resolution with uncertainty <25% between land ecosystems and atmosphere and between ocean ecosystems and atmosphere.
- Ecosystem fluxes, over time, are key to understanding earths carbon and emissions cycles for reporting at all levels of Australian government.
- Cal/Val for this on the ground is already provided (for decades) by the TERN OzFlux network across Australia (https://www.ozflux.org.au/)
Hydrology
H1c: Quantify rates of snow accumulation, snowmelt, ice melt, and sublimation from snow and ice worldwide at scales driven by topographic variability.
- We often forget Australia gets snow in our Snowy Mountains and Tablelands, but as it is critical across the globe, it is here equally important to our ecosystems and hydrological climatology. SBG means more accurate snow information for Australia.
Solid Earth
S1a: Measure the pre-, syn-, and posteruption surface deformation and products of Earth’s entire active land volcano inventory at a time scale of days to weeks.
- Locally in Australia we don’t have any active volcanoes, but we do regionally and these impact our climate.
- What we do have is dust emissions that is relevant here, is dust emissions from our desserts and SBG will help us quantify these better.
What can you do ?
- You can join the NASA SBG working group by emailing NASA on : sbg@jpl.nasa.gov
- Get smart on the missions publications by reading them, they are amassing so here is a Google Scholar link: https://scholar.google.com.au/scholar?hl=en&as_sdt=0%2C5&q=NASA+SBG&btnG=
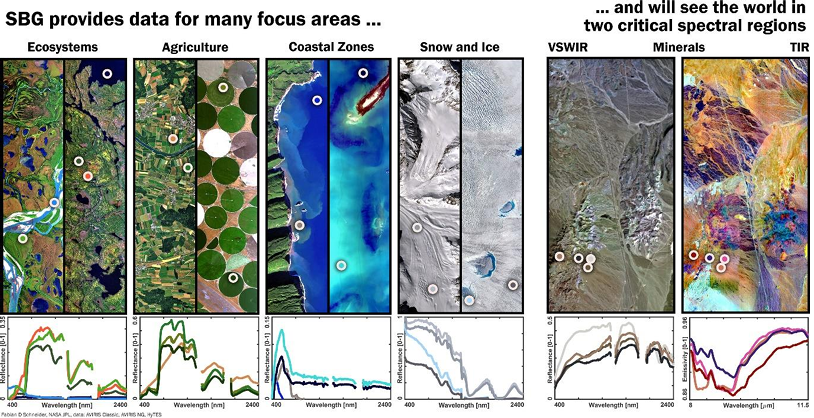